A Guide to Everything You Need to Know About Rasa Chatbot
A Guide to Everything You Need to Know About Rasa Chatbot
Other
19 minutes



A Guide to Everything You Need to Know About Rasa Chatbot
Consider this: You’re in a store, scoping out a new pair of shoes. Suddenly, a sales bot appears on the screen, asking if you need help. You think it looks pretty cool until it opens its mouth. “Hello. I am a virtual assistant. How can I help you today?” Ugh. You close the window and move on. This is the world of chatbots today, and it’s not great. Sure, the technology works. But the experience is anything but human—and that’s a problem. In this guide, we’ll discuss the Rasa Chatbot integration. Rasa is an open-source machine learning framework that allows you to build chatbots with advanced artificial intelligence. Unlike traditional chatbots, which operate on a simple if/then structure, Rasa chatbots use natural language processing to have human-like conversations.
This means when you integrate Rasa chatbots into your business, you can create a more personalised, human-like experience for your customers. Sounds great, right? But is Rasa the right fit for your business? By the end of this guide, you’ll know precisely what Rasa chatbots can do, how they work, and whether they’re the right solution for your business needs. Droxy's AI agent for your business can help you achieve your goals faster by providing you with a clear understanding of Rasa Chatbot's capabilities and how to get started with integration.
Table of Contents
Introduction to Rasa Chatbot

What is Rasa Chatbot?
Rasa Chatbot is an advanced, open-source framework designed to build sophisticated conversational AI assistants that engage in natural, context-aware dialogues with users. Unlike traditional rule-based chatbots that rely on simple keyword matching and scripted responses, Rasa leverages cutting-edge natural language processing (NLP) and machine learning to understand the full context of conversations and respond intelligently in real time.
At its core, Rasa is built on two main components, Rasa NLU (Natural Language Understanding) and Rasa Core. Rasa NLU is responsible for interpreting user input and extracting intents (what the user wants) and entities (key information such as dates, names, or locations). Rasa Core manages the dialogue flow, determining how the chatbot should respond based on the conversation's history, business logic, and extracted information. This modular architecture allows developers to create chatbots that handle complex, multi-turn conversations and remember context across interactions.
Why Rasa Stands Out From Other Chatbot Development Frameworks
One of Rasa’s defining features is its open-source nature. Released under the Apache 2.0 license, Rasa’s codebase is freely accessible, modifiable, and extensible by anyone. This openness has fueled a global community of developers and contributors, driving continuous innovation and improvement. Rasa’s open-source approach contrasts with proprietary chatbot platforms, giving organizations complete control over their data, models, and deployment environments—on-premises or in the cloud.
Who is Using Rasa?
Rasa’s popularity has skyrocketed among both developers and enterprises. It is widely adopted across customer support, healthcare, banking, e-commerce, and more industries. The platform’s flexibility and scalability make it suitable for use cases ranging from simple FAQ bots to complex virtual assistants capable of handling high volumes of conversations and integrating with external systems. As of late 2024, Rasa boasts over 10,000 GitHub stars, more than 500 contributors, and millions of monthly downloads, with users ranging from startups to Fortune 500 companies.
Why Developers Love Rasa
Developers are drawn to Rasa for its powerful customization options, robust NLU capabilities, and ability to integrate with various messaging channels and voice assistants. For example, a Rasa-powered chatbot can be deployed on web applications like Facebook Messenger, Slack, WhatsApp, or voice platforms like Amazon Alexa and Google Assistant. The framework supports text and voice-based interactions, making it versatile for modern conversational AI needs.
Related Reading
Key Features of Rasa Chatbot

Customizable Conversational Flows and Dialogue Management
Rasa excels in managing complex, multi-turn conversations through its modular architecture, which consists of two main components: Rasa NLU (Natural Language Understanding) and Rasa Core (Dialogue Management). Rasa NLU interprets user input, extracting intents and entities to understand the user's wants and the key information provided. Rasa Core manages the flow of the conversation, allowing developers to define “stories” or conversation paths and train the bot to make decisions based on conversation history and context. Developers can build dynamic, context-aware chatbots that remember previous user inputs and provide personalized responses, making interactions feel more natural and human-like.
Integration with Machine Learning Tools
Rasa is designed to be highly extensible and integrates smoothly with popular machine learning libraries such as spaCy, TensorFlow, and PyTorch. This integration enables developers to leverage powerful NLP models for intent recognition, entity extraction, and contextual understanding. Rasa’s machine learning-driven approach allows the chatbot to improve over time as it is exposed to more conversational data, making it adaptable to evolving user needs.
Support for Multiple Languages and Platforms
Rasa supports the development of multilingual chatbots, making it a strong choice for businesses operating in global markets. Developers can train Rasa models on datasets in various languages, ensuring accurate understanding and response generation for diverse user bases. Rasa bots can be deployed across multiple messaging platforms, including Facebook Messenger, Slack, WhatsApp, Microsoft Teams, Telegram, and more, allowing organizations to reach users on their preferred channels.
On-Premises or Cloud Deployment for Full Data Control
One of Rasa’s standout features is its flexible deployment options. Organizations can deploy Rasa chatbots on-premises, in a private cloud, or via public cloud services, depending on their security and compliance requirements. This flexibility ensures businesses retain complete control over their conversational data, which is crucial for industries with strict privacy or regulatory standards.
Extensible with Custom Actions, APIs, and Business Logic
Rasa allows developers to create custom actions that execute complex tasks, such as querying databases, calling external APIs, or integrating with business systems. These custom actions enable chatbots to go beyond static responses and perform real-time operations based on user requests. Developers can define domain-specific logic, configure the bot’s domain (including intents, entities, and responses), and extend the chatbot’s capabilities to fit unique business needs.
Advantages of Using Rasa
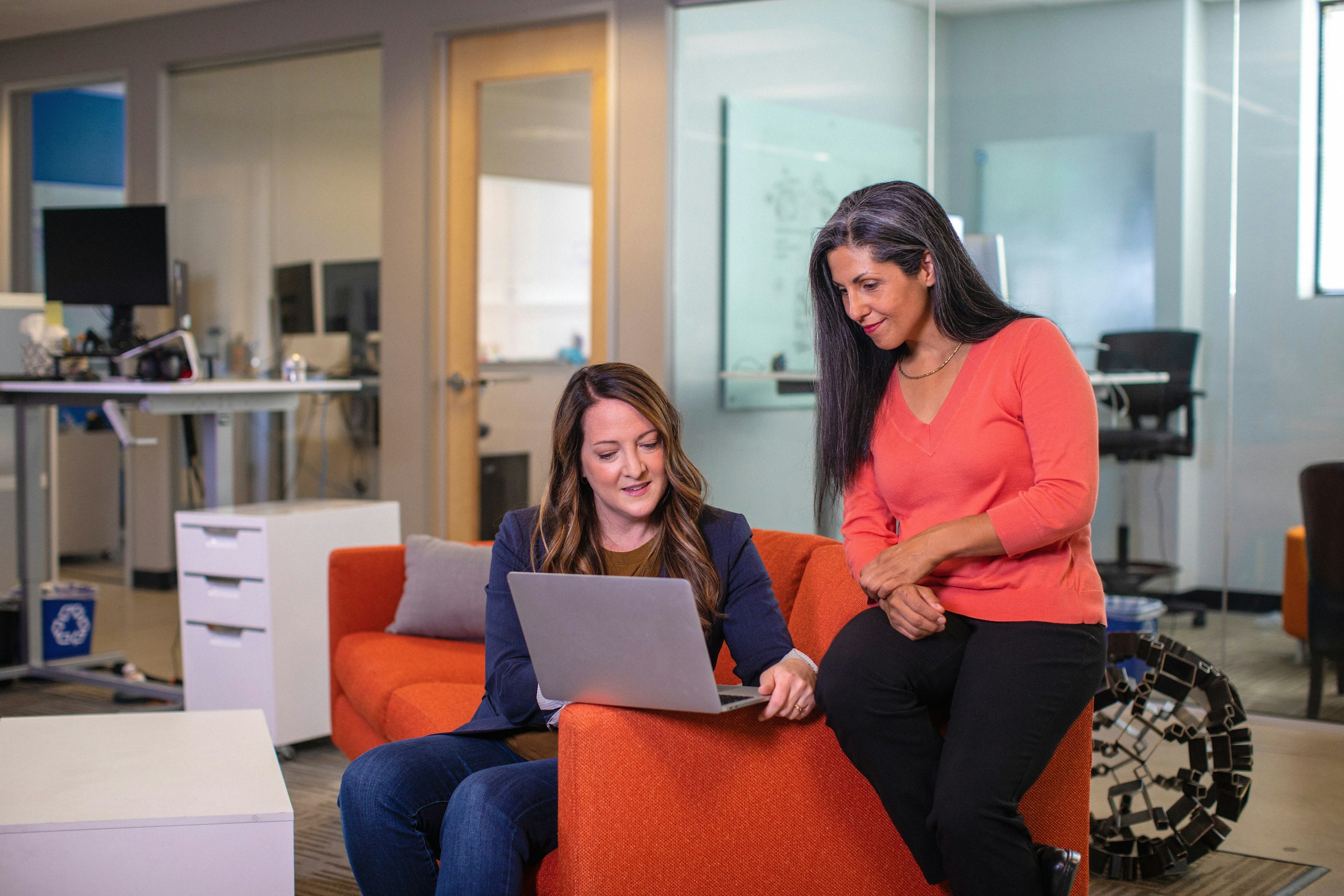
1. High Flexibility and Customization for Complex Use Cases
Rasa is known for its exceptional flexibility, allowing developers to design chatbots that handle intricate conversational flows, multi-turn dialogues, and industry-specific requirements. Its modular architecture enables users to define custom intents, entities, and dialogue paths, making it possible to build assistants tailored to unique business processes, whether for customer support, sales automation, appointment scheduling, or other advanced use cases. Developers can extend Rasa with custom actions, integrate external APIs, and implement domain-specific logic, ensuring the chatbot fits smoothly into existing workflows.
2. Open-Source and Free to Use, with a Large Developer Community
One of Rasa’s most significant advantages is its open-source nature. The entire codebase is freely accessible, allowing organizations to inspect, modify, and enhance the platform. This transparency reduces vendor lock-in and fosters a large global developer community contributing to continuous innovation and improvement. The open-source model means no licensing fees, making Rasa a cost-effective solution for startups and large enterprises. Community forums, documentation, and pre-built components further accelerate development and troubleshooting.
3. Machine Learning-Based Approach for Improved Conversation Quality Over Time
Unlike rule-based chatbots, Rasa leverages advanced machine learning and natural language processing (NLP) to understand user intent and context. Its learning-based approach allows the chatbot to adapt and improve as it interacts with more users, resulting in more accurate and relevant responses. Rasa’s dialogue management system can handle unexpected conversation turns, learn from real-world data, and maintain context, enabling chatbots to provide personalized and dynamic interactions. This adaptability is crucial for delivering high-quality customer experiences in rapidly changing environments.
4. Scalable to Handle Large Volumes of Conversations
Rasa is designed with scalability, making it suitable for organizations that expect high traffic and need to automate large volumes of customer interactions. Its architecture supports deployment across distributed systems, cloud environments, or on-premises infrastructure, ensuring reliable performance even as user demand grows. This scalability allows businesses to start small and expand their chatbot capabilities as their needs evolve, without worrying about hitting platform limitations.
5. Supports Both Text and Voice-Based Assistants
Another notable advantage of Rasa is its support for text and voice-based conversational interfaces. Developers can build assistants operating across messaging platforms (such as Facebook Messenger, Slack, WhatsApp, and more) and voice channels (like Alexa or Google Assistant), enabling omnichannel customer engagement. This versatility allows organizations to meet users wherever they are and deliver consistent, high-quality experiences across all touchpoints.
Disadvantages and Limitations of Using Rasa

1. Understanding Rasa's Steep Learning Curve
One of Rasa's biggest disadvantages is its steep learning curve. Developing chatbots with Rasa requires a solid understanding of programming, primarily Python, and foundational knowledge of machine learning concepts. Unlike many plug-and-play chatbot builders, Rasa is not designed for users seeking a drag-and-drop interface or low-code solutions. Instead, it expects users to write and maintain code, configure pipelines, and understand the intricacies of intent classification, entity extraction, and dialogue management. This technical barrier can be daunting for beginners or teams without prior experience in AI or software development.
2. Rasa Is More Suited for Developers Than Non-Technical Users
Developers or technical teams best leverage Rasa’s rich feature set and customization options. Non-technical users, such as marketers or business analysts, may find it challenging to set up, train, and maintain a Rasa chatbot without significant support from developers. While the platform offers extensive documentation and a supportive community, the need for coding and system configuration makes Rasa less accessible to those without a technical background. In contrast, other chatbot platforms offer more intuitive interfaces and guided workflows, making them more suitable for business users who want to deploy conversational agents quickly.
3. Initial Setup and Training Can Be Time Consuming
Setting up a Rasa chatbot involves several time-intensive steps, including environment configuration, data preparation, model training, and integration with messaging channels or business systems. Rasa does not provide ready-made templates or instant deployment options, unlike pre-built SaaS chatbot solutions. Users must invest time defining intents, entities, stories, and custom actions and training and testing the models to ensure accurate performance. Additionally, ongoing maintenance such as updating training data and retraining models to improve accuracy requires continuous effort and technical oversight.
4. Resource Requirements for Rasa
Because Rasa is typically deployed on-premises or in private cloud environments, organizations are responsible for managing their infrastructure, which includes provisioning servers, handling updates, and ensuring security. This adds another layer of complexity and operational overhead compared to fully managed cloud chatbot services.
5. Limited Out-of-the-Box Functionality
While Rasa’s open-source nature allows for deep customization, it also means that many features available by default in commercial platforms, such as analytics dashboards, visual flow builders, or pre-configured integration,s must be built or integrated separately.
6. Emotionless and Lacks Human Touch
Like most chatbots, Rasa-based assistants can struggle with understanding nuanced emotions or complex, context-rich queries, which may lead to user frustration in sensitive scenarios.
Related Reading
Rasa Chatbot vs. Droxy

Rasa Chatbot: The Developer-Friendly Conversational AI Framework
Rasa Chatbot is an open-source framework for developers and technical teams looking to create fully customizable and complex conversational agents. While Rasa provides extensive flexibility and control, it requires programming skills (particularly in Python) and knowledge of machine learning concepts to build, train, and deploy its models. Rasa’s strength lies in its adaptability: developers can define custom intents, entities, dialogue flows, and integrate sophisticated machine learning pipelines. The framework also supports on-premises deployment, giving organizations complete control over data and compliance, which is crucial for industries with strict regulatory requirements. Rasa also integrates with various messaging platforms and backend systems, making it suitable for enterprises with complex, bespoke needs.
Droxy: The Fast and Easy No-Code Chatbot Solution
In contrast, Droxy is a no-code AI agent platform that democratizes chatbot creation for businesses of all sizes and technical backgrounds. Its intuitive interface allows users to rapidly build, customize, and deploy AI chatbots without writing code. Droxy transforms static content such as PDFs, videos, and websites into interactive chatbots that engage customers naturally. With Droxy, non-technical users can create and manage chatbots independently, reducing reliance on IT or AI specialists. This lowers the total cost of ownership for organizations seeking to leverage conversational AI to improve customer experience.
Rasa and Droxy Have Very Different Target Audiences
Rasa Chatbot is ideal for organizations with strong technical teams seeking deep customization, complete control over data, and the ability to build complex conversational AI tailored to unique workflows. It is the go-to for enterprises with specific compliance and scalability demands. On the other hand, Droxy provides a powerful, no-code alternative designed for businesses that prioritize speed, ease of use, and broad omnichannel engagement. Its user-friendly platform enables fast chatbot deployment without technical hurdles, making it perfect for companies looking to enhance customer experience and operational efficiency quickly. For businesses seeking a balance of customization and accessibility, Droxy offers a modern, scalable solution that reduces complexity and accelerates time to value in conversational AI.
Related Reading
Botsonic Alternative
Landbot Alternatives
Create an AI Agent for Your Business for Free within 5 Minutes
Droxy's AI chatbot platform helps businesses create custom chatbots to streamline customer service and support. The AI agent engages with customers in natural, human-like conversations, answering questions and solving problems. If the bot encounters a query it can't handle, it smoothly transitions the interaction to a human agent for further assistance. Droxy's chatbot can help companies boost sales, improve customer satisfaction, and reduce operational costs.
A Guide to Everything You Need to Know About Rasa Chatbot
Consider this: You’re in a store, scoping out a new pair of shoes. Suddenly, a sales bot appears on the screen, asking if you need help. You think it looks pretty cool until it opens its mouth. “Hello. I am a virtual assistant. How can I help you today?” Ugh. You close the window and move on. This is the world of chatbots today, and it’s not great. Sure, the technology works. But the experience is anything but human—and that’s a problem. In this guide, we’ll discuss the Rasa Chatbot integration. Rasa is an open-source machine learning framework that allows you to build chatbots with advanced artificial intelligence. Unlike traditional chatbots, which operate on a simple if/then structure, Rasa chatbots use natural language processing to have human-like conversations.
This means when you integrate Rasa chatbots into your business, you can create a more personalised, human-like experience for your customers. Sounds great, right? But is Rasa the right fit for your business? By the end of this guide, you’ll know precisely what Rasa chatbots can do, how they work, and whether they’re the right solution for your business needs. Droxy's AI agent for your business can help you achieve your goals faster by providing you with a clear understanding of Rasa Chatbot's capabilities and how to get started with integration.
Table of Contents
Introduction to Rasa Chatbot

What is Rasa Chatbot?
Rasa Chatbot is an advanced, open-source framework designed to build sophisticated conversational AI assistants that engage in natural, context-aware dialogues with users. Unlike traditional rule-based chatbots that rely on simple keyword matching and scripted responses, Rasa leverages cutting-edge natural language processing (NLP) and machine learning to understand the full context of conversations and respond intelligently in real time.
At its core, Rasa is built on two main components, Rasa NLU (Natural Language Understanding) and Rasa Core. Rasa NLU is responsible for interpreting user input and extracting intents (what the user wants) and entities (key information such as dates, names, or locations). Rasa Core manages the dialogue flow, determining how the chatbot should respond based on the conversation's history, business logic, and extracted information. This modular architecture allows developers to create chatbots that handle complex, multi-turn conversations and remember context across interactions.
Why Rasa Stands Out From Other Chatbot Development Frameworks
One of Rasa’s defining features is its open-source nature. Released under the Apache 2.0 license, Rasa’s codebase is freely accessible, modifiable, and extensible by anyone. This openness has fueled a global community of developers and contributors, driving continuous innovation and improvement. Rasa’s open-source approach contrasts with proprietary chatbot platforms, giving organizations complete control over their data, models, and deployment environments—on-premises or in the cloud.
Who is Using Rasa?
Rasa’s popularity has skyrocketed among both developers and enterprises. It is widely adopted across customer support, healthcare, banking, e-commerce, and more industries. The platform’s flexibility and scalability make it suitable for use cases ranging from simple FAQ bots to complex virtual assistants capable of handling high volumes of conversations and integrating with external systems. As of late 2024, Rasa boasts over 10,000 GitHub stars, more than 500 contributors, and millions of monthly downloads, with users ranging from startups to Fortune 500 companies.
Why Developers Love Rasa
Developers are drawn to Rasa for its powerful customization options, robust NLU capabilities, and ability to integrate with various messaging channels and voice assistants. For example, a Rasa-powered chatbot can be deployed on web applications like Facebook Messenger, Slack, WhatsApp, or voice platforms like Amazon Alexa and Google Assistant. The framework supports text and voice-based interactions, making it versatile for modern conversational AI needs.
Related Reading
Key Features of Rasa Chatbot

Customizable Conversational Flows and Dialogue Management
Rasa excels in managing complex, multi-turn conversations through its modular architecture, which consists of two main components: Rasa NLU (Natural Language Understanding) and Rasa Core (Dialogue Management). Rasa NLU interprets user input, extracting intents and entities to understand the user's wants and the key information provided. Rasa Core manages the flow of the conversation, allowing developers to define “stories” or conversation paths and train the bot to make decisions based on conversation history and context. Developers can build dynamic, context-aware chatbots that remember previous user inputs and provide personalized responses, making interactions feel more natural and human-like.
Integration with Machine Learning Tools
Rasa is designed to be highly extensible and integrates smoothly with popular machine learning libraries such as spaCy, TensorFlow, and PyTorch. This integration enables developers to leverage powerful NLP models for intent recognition, entity extraction, and contextual understanding. Rasa’s machine learning-driven approach allows the chatbot to improve over time as it is exposed to more conversational data, making it adaptable to evolving user needs.
Support for Multiple Languages and Platforms
Rasa supports the development of multilingual chatbots, making it a strong choice for businesses operating in global markets. Developers can train Rasa models on datasets in various languages, ensuring accurate understanding and response generation for diverse user bases. Rasa bots can be deployed across multiple messaging platforms, including Facebook Messenger, Slack, WhatsApp, Microsoft Teams, Telegram, and more, allowing organizations to reach users on their preferred channels.
On-Premises or Cloud Deployment for Full Data Control
One of Rasa’s standout features is its flexible deployment options. Organizations can deploy Rasa chatbots on-premises, in a private cloud, or via public cloud services, depending on their security and compliance requirements. This flexibility ensures businesses retain complete control over their conversational data, which is crucial for industries with strict privacy or regulatory standards.
Extensible with Custom Actions, APIs, and Business Logic
Rasa allows developers to create custom actions that execute complex tasks, such as querying databases, calling external APIs, or integrating with business systems. These custom actions enable chatbots to go beyond static responses and perform real-time operations based on user requests. Developers can define domain-specific logic, configure the bot’s domain (including intents, entities, and responses), and extend the chatbot’s capabilities to fit unique business needs.
Advantages of Using Rasa
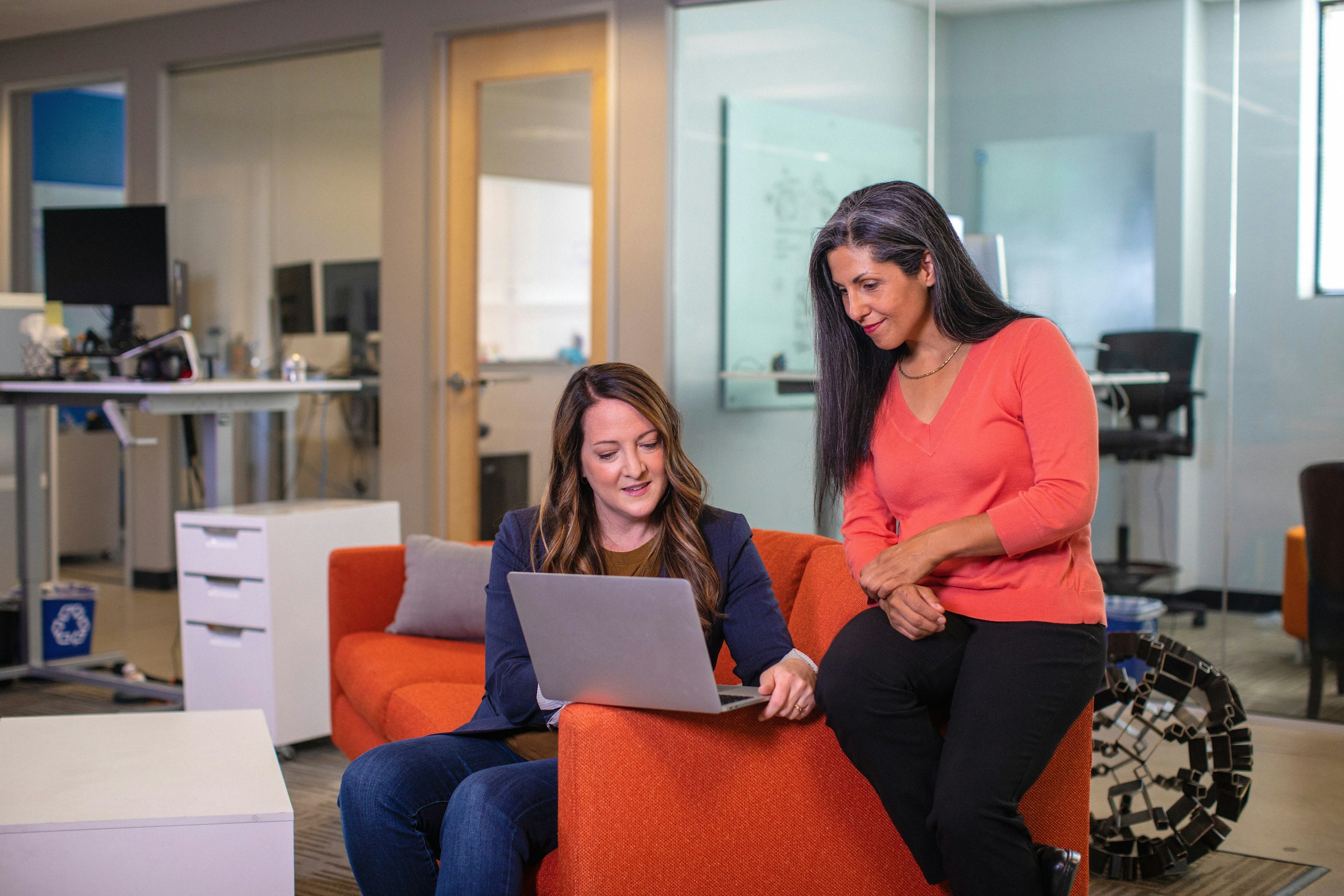
1. High Flexibility and Customization for Complex Use Cases
Rasa is known for its exceptional flexibility, allowing developers to design chatbots that handle intricate conversational flows, multi-turn dialogues, and industry-specific requirements. Its modular architecture enables users to define custom intents, entities, and dialogue paths, making it possible to build assistants tailored to unique business processes, whether for customer support, sales automation, appointment scheduling, or other advanced use cases. Developers can extend Rasa with custom actions, integrate external APIs, and implement domain-specific logic, ensuring the chatbot fits smoothly into existing workflows.
2. Open-Source and Free to Use, with a Large Developer Community
One of Rasa’s most significant advantages is its open-source nature. The entire codebase is freely accessible, allowing organizations to inspect, modify, and enhance the platform. This transparency reduces vendor lock-in and fosters a large global developer community contributing to continuous innovation and improvement. The open-source model means no licensing fees, making Rasa a cost-effective solution for startups and large enterprises. Community forums, documentation, and pre-built components further accelerate development and troubleshooting.
3. Machine Learning-Based Approach for Improved Conversation Quality Over Time
Unlike rule-based chatbots, Rasa leverages advanced machine learning and natural language processing (NLP) to understand user intent and context. Its learning-based approach allows the chatbot to adapt and improve as it interacts with more users, resulting in more accurate and relevant responses. Rasa’s dialogue management system can handle unexpected conversation turns, learn from real-world data, and maintain context, enabling chatbots to provide personalized and dynamic interactions. This adaptability is crucial for delivering high-quality customer experiences in rapidly changing environments.
4. Scalable to Handle Large Volumes of Conversations
Rasa is designed with scalability, making it suitable for organizations that expect high traffic and need to automate large volumes of customer interactions. Its architecture supports deployment across distributed systems, cloud environments, or on-premises infrastructure, ensuring reliable performance even as user demand grows. This scalability allows businesses to start small and expand their chatbot capabilities as their needs evolve, without worrying about hitting platform limitations.
5. Supports Both Text and Voice-Based Assistants
Another notable advantage of Rasa is its support for text and voice-based conversational interfaces. Developers can build assistants operating across messaging platforms (such as Facebook Messenger, Slack, WhatsApp, and more) and voice channels (like Alexa or Google Assistant), enabling omnichannel customer engagement. This versatility allows organizations to meet users wherever they are and deliver consistent, high-quality experiences across all touchpoints.
Disadvantages and Limitations of Using Rasa

1. Understanding Rasa's Steep Learning Curve
One of Rasa's biggest disadvantages is its steep learning curve. Developing chatbots with Rasa requires a solid understanding of programming, primarily Python, and foundational knowledge of machine learning concepts. Unlike many plug-and-play chatbot builders, Rasa is not designed for users seeking a drag-and-drop interface or low-code solutions. Instead, it expects users to write and maintain code, configure pipelines, and understand the intricacies of intent classification, entity extraction, and dialogue management. This technical barrier can be daunting for beginners or teams without prior experience in AI or software development.
2. Rasa Is More Suited for Developers Than Non-Technical Users
Developers or technical teams best leverage Rasa’s rich feature set and customization options. Non-technical users, such as marketers or business analysts, may find it challenging to set up, train, and maintain a Rasa chatbot without significant support from developers. While the platform offers extensive documentation and a supportive community, the need for coding and system configuration makes Rasa less accessible to those without a technical background. In contrast, other chatbot platforms offer more intuitive interfaces and guided workflows, making them more suitable for business users who want to deploy conversational agents quickly.
3. Initial Setup and Training Can Be Time Consuming
Setting up a Rasa chatbot involves several time-intensive steps, including environment configuration, data preparation, model training, and integration with messaging channels or business systems. Rasa does not provide ready-made templates or instant deployment options, unlike pre-built SaaS chatbot solutions. Users must invest time defining intents, entities, stories, and custom actions and training and testing the models to ensure accurate performance. Additionally, ongoing maintenance such as updating training data and retraining models to improve accuracy requires continuous effort and technical oversight.
4. Resource Requirements for Rasa
Because Rasa is typically deployed on-premises or in private cloud environments, organizations are responsible for managing their infrastructure, which includes provisioning servers, handling updates, and ensuring security. This adds another layer of complexity and operational overhead compared to fully managed cloud chatbot services.
5. Limited Out-of-the-Box Functionality
While Rasa’s open-source nature allows for deep customization, it also means that many features available by default in commercial platforms, such as analytics dashboards, visual flow builders, or pre-configured integration,s must be built or integrated separately.
6. Emotionless and Lacks Human Touch
Like most chatbots, Rasa-based assistants can struggle with understanding nuanced emotions or complex, context-rich queries, which may lead to user frustration in sensitive scenarios.
Related Reading
Rasa Chatbot vs. Droxy

Rasa Chatbot: The Developer-Friendly Conversational AI Framework
Rasa Chatbot is an open-source framework for developers and technical teams looking to create fully customizable and complex conversational agents. While Rasa provides extensive flexibility and control, it requires programming skills (particularly in Python) and knowledge of machine learning concepts to build, train, and deploy its models. Rasa’s strength lies in its adaptability: developers can define custom intents, entities, dialogue flows, and integrate sophisticated machine learning pipelines. The framework also supports on-premises deployment, giving organizations complete control over data and compliance, which is crucial for industries with strict regulatory requirements. Rasa also integrates with various messaging platforms and backend systems, making it suitable for enterprises with complex, bespoke needs.
Droxy: The Fast and Easy No-Code Chatbot Solution
In contrast, Droxy is a no-code AI agent platform that democratizes chatbot creation for businesses of all sizes and technical backgrounds. Its intuitive interface allows users to rapidly build, customize, and deploy AI chatbots without writing code. Droxy transforms static content such as PDFs, videos, and websites into interactive chatbots that engage customers naturally. With Droxy, non-technical users can create and manage chatbots independently, reducing reliance on IT or AI specialists. This lowers the total cost of ownership for organizations seeking to leverage conversational AI to improve customer experience.
Rasa and Droxy Have Very Different Target Audiences
Rasa Chatbot is ideal for organizations with strong technical teams seeking deep customization, complete control over data, and the ability to build complex conversational AI tailored to unique workflows. It is the go-to for enterprises with specific compliance and scalability demands. On the other hand, Droxy provides a powerful, no-code alternative designed for businesses that prioritize speed, ease of use, and broad omnichannel engagement. Its user-friendly platform enables fast chatbot deployment without technical hurdles, making it perfect for companies looking to enhance customer experience and operational efficiency quickly. For businesses seeking a balance of customization and accessibility, Droxy offers a modern, scalable solution that reduces complexity and accelerates time to value in conversational AI.
Related Reading
Botsonic Alternative
Landbot Alternatives
Create an AI Agent for Your Business for Free within 5 Minutes
Droxy's AI chatbot platform helps businesses create custom chatbots to streamline customer service and support. The AI agent engages with customers in natural, human-like conversations, answering questions and solving problems. If the bot encounters a query it can't handle, it smoothly transitions the interaction to a human agent for further assistance. Droxy's chatbot can help companies boost sales, improve customer satisfaction, and reduce operational costs.
🚀
Powered by Droxy
Turn every interaction into a conversion
Customer facing AI agents that engage, convert, and support so you can scale what matters.
✨
Learn more
Recent posts
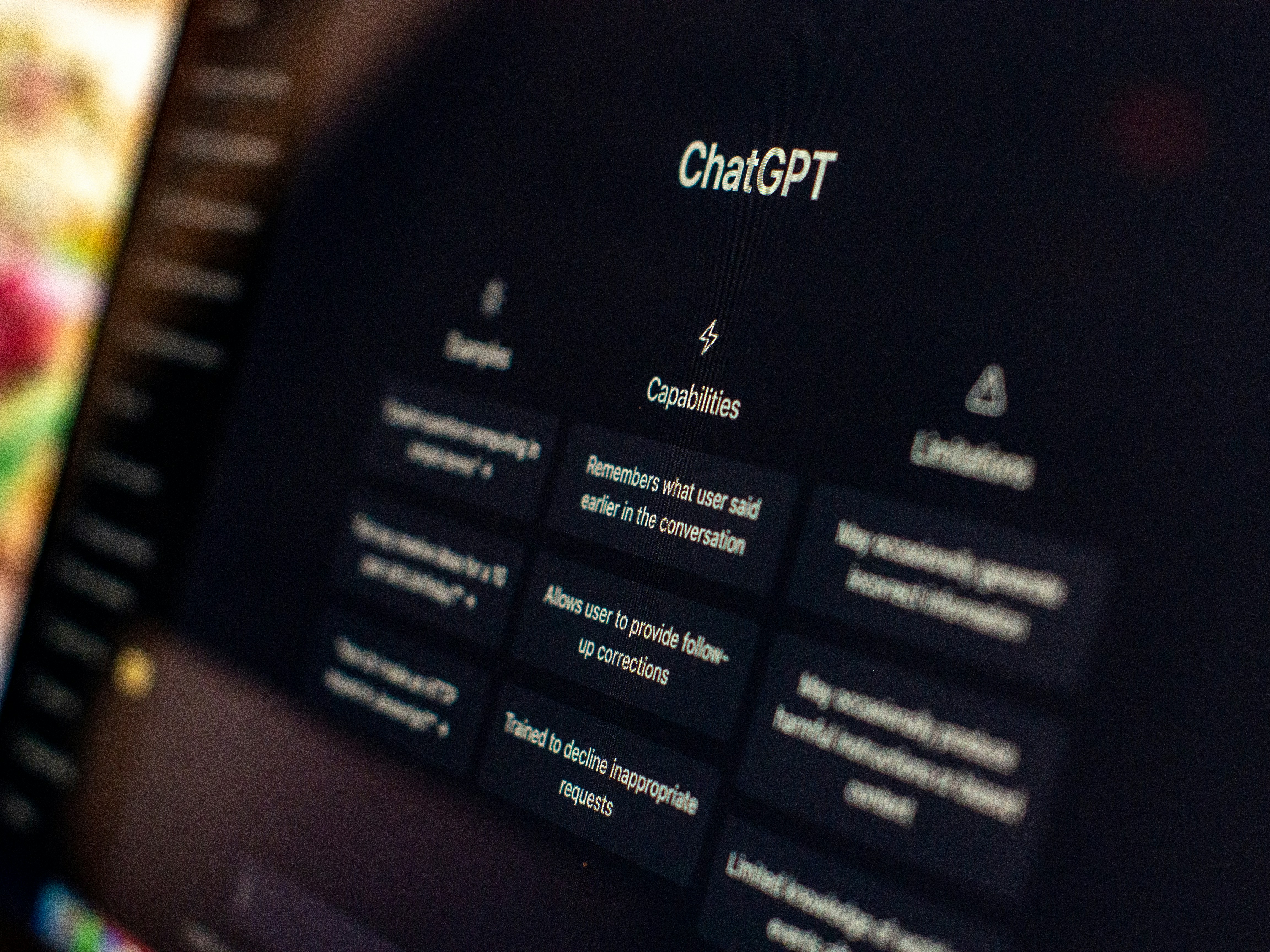
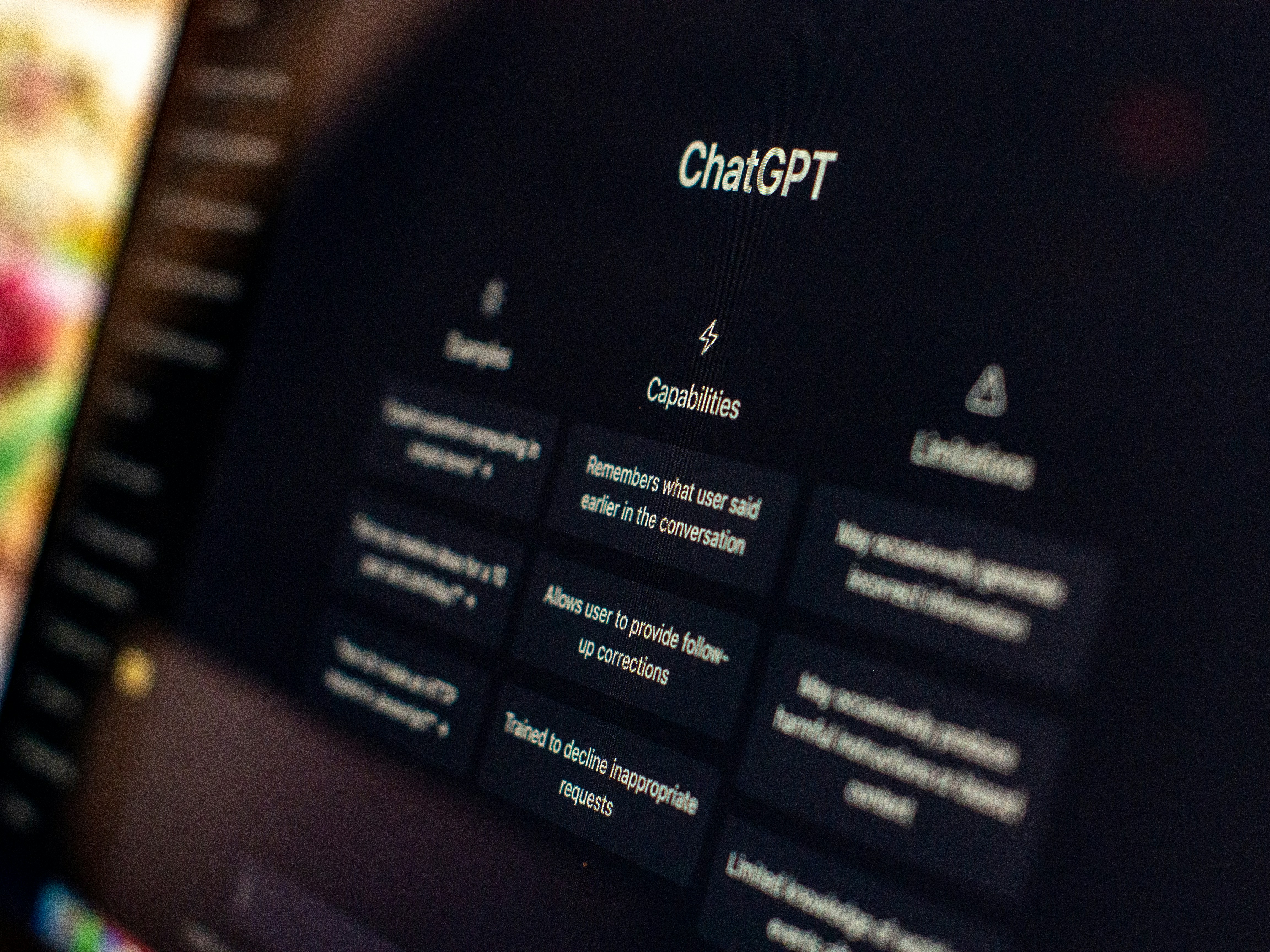
Other
25 min
Top 10 Chatbase Alternatives You Should Know in 2025
Tired of Chatbase’s limits? Discover powerful Chatbase alternatives that offer deeper analytics, better UX, and custom AI workflows.
Read more


Other
26 min
Top 7 Botpress Alternatives You Should Know in 2025
Explore top Botpress alternatives like Droxy, Voiceflow, Chatfuel, and Intercom to find the best AI chatbot platforms for your needs.
Read more


Other
16 minutes
The Best ManyChat Alternative in 2025
Looking for a ManyChat alternative in 2025? Discover top options with better features, pricing, and ease of use.
Read more
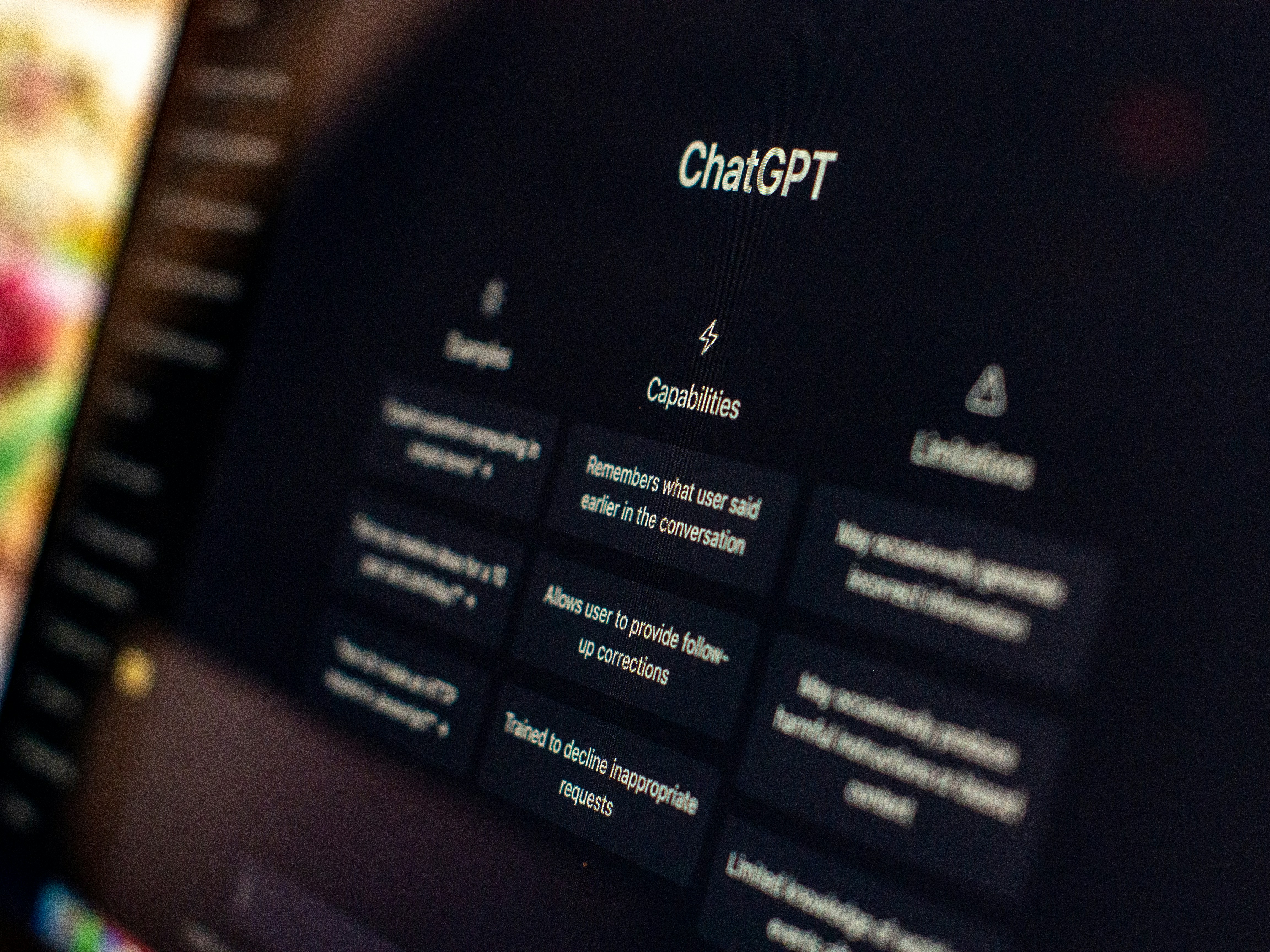
Other
25 min
Top 10 Chatbase Alternatives You Should Know in 2025
Tired of Chatbase’s limits? Discover powerful Chatbase alternatives that offer deeper analytics, better UX, and custom AI workflows.
Read more

Other
26 min
Top 7 Botpress Alternatives You Should Know in 2025
Explore top Botpress alternatives like Droxy, Voiceflow, Chatfuel, and Intercom to find the best AI chatbot platforms for your needs.
Read more